Nvidia's market value fluctuates at high levels, and the industry is increasingly focusing on AI chips beyond GPUs (Graphics Processing Units).
Recently, two highly anticipated funding news in the AI chip sector are both related to ASIC chips (Application-Specific Integrated Circuits). It is reported that AI chip startup Groq will be valued at $2.5 billion in a new round of financing, and another startup, Etched, has completed a $120 million financing round; both are working on ASICs. The GPU giant Nvidia also seems to be considering the impact of market competition, with news in early this year that Nvidia is contemplating entering the ASIC field.
Relatively speaking, GPUs are more versatile and have a more mature software ecosystem, capable of running various algorithms, while ASICs have weaker generality but potentially stronger hardware performance, possibly only capable of running a subset of algorithms. In addition to AI startups and cloud vendors steering clear of Nvidia's edge to focus on ASICs, reporters have also learned that computing power providers are considering the use of FPGA (Field-Programmable Gate Array) chips suitable for edge computing. Demand for computing power is seeking more diverse AI chip solutions.
Advertisement
These AI chip companies are seen as competitors to Nvidia, so to what extent can these AI chips encroach on Nvidia's GPU market?
The business of ASICs, both on and off the table
The highly anticipated financing of ASIC startups and the low-profile layout of cloud giants, both on and off the table, ASICs are launching an offensive against GPUs.
Among the two startups that recently announced financing news, Groq, founded by one of the inventors of Google's TPU (Tensor Processing Unit), Jonathan Ross, launched the ASIC chip LPU (Language Processing Unit) in February this year, claiming that the LPU's inference performance is 10 times that of Nvidia's GPU, with a cost only one-tenth of it. Etched, on the other hand, launched the Sohu chip in June, optimizing the Transformer (the architecture based on which mainstream large language models are built) and fixing it on the chip, claiming that the performance of a server integrated with 8 Sohus is equivalent to 160 H100 GPUs.
Many cloud giants also develop their own ASIC chips, including Google, Microsoft, Meta, etc., with Google's self-used ASIC shipments already substantial. According to the latest data from market research institution TechInsights, in 2023, among data center accelerator manufacturers, Google TPU shipments reached 2 million units, Nvidia 3.8 million units, and other chips 500,000 units. In 2023, Google has become the third-largest data center processor designer, and in the fourth quarter of 2023, Nvidia's share of the data center processor market exceeded 50%, with Google's market share ranking third, approaching the market share of the second-place Intel.
Some AI custom chip manufacturers have thus become invisible winners. Google collaborated with Broadcom to develop TPU. In the first quarter of the 2024 fiscal year, Broadcom's revenue was $11.961 billion, a year-on-year increase of 34%. Broadcom CEO Hock Tan stated that in the second quarter, Broadcom's revenue from AI products reached a record $3.1 billion, with artificial intelligence demand being a significant factor in boosting performance.
From the beginning of this year to July 8th local time, Broadcom's market value has increased by more than $200 billion. Another major AI custom chip manufacturer, Marvell, saw a significant growth in its AI custom chip business in the first quarter of the 2025 fiscal year, which ended on May 4th, driving its data center business revenue to grow by 87%.In principle, Etched has previously stated that CPUs and GPUs, as general-purpose chips, need to adapt to different AI architectures, hence most of their computing power is not utilized for AI-related computations. It is estimated that only 3.3% of the transistors in the H100 are dedicated to matrix multiplication. Non-general-purpose chips have fewer such concerns. Sohu has also managed to allocate more space for computation by reducing memory space, among other methods. Groq has specifically designed chips for processing large language model tasks and has combined them with near-memory computing technology to enhance computational performance. At a 14nm process, Groq's large model generation speed reaches nearly 500 tokens per second, surpassing the 40 tokens per second of GPU-driven GPT-3.5.
If better-performing hardware can be used in AI scenarios, it implies that the same amount of computation can be completed with lower energy consumption. Moreover, Nvidia recorded a gross margin as high as 78.4% in the first fiscal quarter of 2025, which ended on April 28th, indicating their undeniable control over pricing power. If AI computing demanders can develop or purchase ASIC chips, they might be able to reduce the hardware prices of AI chips as well.
Although migrating from a mature GPU ecosystem to an ASIC ecosystem requires costs, and the latter's software ecosystem is not as mature as the GPU's, the industry is already considering replacing some GPU computing power with ASICs. In addition to cloud vendors like Google using ASIC chips for large model training, market analysis mainly suggests that ASICs can replace GPUs in model inference scenarios.
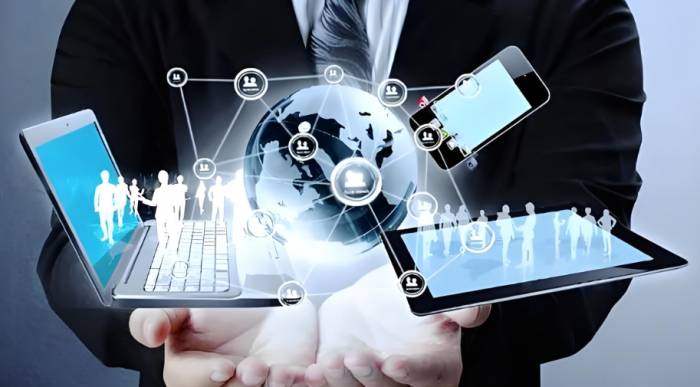
"Enterprises need to prove the rationality of their expenditures and returns; they will not be able to 'luxuriate' in using expensive GPUs to meet all AI needs. Enterprises will still use GPUs because they are still needed for a large number of parallelized general-use cases, but for other needs, ASICs running in the right environment will be a better choice because their purchase cost is lower, and there will be more ASIC designs to meet specific needs," Owen Rogers predicts that model training will still be carried out on GPUs because they are more flexible for different models, while inference may increasingly use low-power ASICs.
A report released by McKinsey in March also indicated that the current mainstream high-performance next-generation AI servers use a combination of 2 CPUs and 8 GPUs, with inference workloads running on infrastructure similar to that used for training. As AI workloads are expected to shift mainly towards inference in the future, the workloads will be primarily handled by specialized hardware. By 2030, AI accelerators equipped with ASIC chips will handle most AI workloads because ASICs perform better in specific AI tasks.
Who else is a potential competitor to GPUs?
Apart from ASICs, other chip architectures are also trying to enter the market. FPGAs have previously been considered suitable for edge computing due to their good hardware flexibility, latency performance, and lower power consumption. The two main FPGA companies are Xilinx, in which AMD has a stake, and Altera, a subsidiary of Intel. Currently, FPGAs have shown a trend of penetrating the large model domain. Domestically, Wuwen Xingqiong, Tsinghua University, and Shanghai Jiao Tong University jointly proposed a lightweight deployment process for large models on FPGAs called FlightLLM in January. For the first time, they achieved efficient inference of LLaMA2-7B on a single Xilinx U280 FPGA.
"When not running large models, the cost difference between GPUs and FPGAs is not too significant, but the gap widens when running large models because the model parameters are larger, and the required number of chip cards increases exponentially," a computational chip technician told a reporter. The power consumption of an FPGA is about tens of watts when in use, while a GPU has a standby power consumption of tens of watts and can reach over 300 watts during inference, which means that the cost of using an FPGA integrated machine is lower compared to a GPU.
The technician stated that compared to GPUs, which can be used for both training and inference, FPGAs are relatively more suitable for large model edge inference. It is expected that in the future, they will form a certain substitution for GPUs in inference scenarios. The computational platform company where he works is already adapting to FPGAs but has not yet officially launched commercial products. However, the technician mentioned that using FPGAs also has disadvantages. These chips are customized, have a high development difficulty, and require reprogramming. The iteration cycle will gradually shorten after running some large models initially. Nowadays, many industry customers are interested in non-GPU computational solutions, and many are inquiring, but not many are actually using them.
Other innovative chip forms are also eyeing the large model market. In June of this year, there were reports that AI chip company Cerebras had secretly submitted IPO documents to securities regulatory authorities. Contrary to some ordinary high-process chips that are becoming smaller and smaller, Cerebras' approach is to make the chips larger while using high-process technology. In 2019, Cerebras launched the "world's largest chip," the wafer-scale chip WSE, which integrated 400,000 AI cores and 1.2 trillion transistors on an area of 46,225 mm². In March of this year, they introduced the third-generation wafer-scale chip WSE-3, whose core count is 52 times that of Nvidia's H100.Owen Rogers informed the press that Cerebras has all components on a single wafer, which minimizes the distance between multiple cores and memory, reducing latency and increasing bandwidth. For AI workloads requiring substantial parallel computation and large memory, this can significantly enhance performance and reduce power consumption. The key features of Cerebras stem from its chip design methodology. However, in addition to designing, shipping, and selling hardware, Cerebras also needs to adjust existing open-source frameworks to adapt to its system, reducing difficulties for new customers when porting existing models.
From a general perspective, whether it's Cerebras, Etched, or some FPGA chips, the trend towards more specialized or customized chips benefits the more efficient operation of large models on chips, but it also faces some challenges in development or adaptation. What kind of new chips can carve out a new path, the outcome remains to be seen. Gavin Uberti, the co-founder and CEO of Etched, which makes Transformer chips, once stated that the company is taking a gamble—if Transformers are no longer adopted, the company will fail, but if Transformers continue to be used, the company could become one of the largest in history.
Faced with the challenges of more specialized chips, GPUs are not standing still either. Journalists learned that disrupting their own versatility and moving towards specialization is also a possible path. Some new technologies are also expected to be used to overcome the limitations of GPUs and meet the challenges from other chips.
A senior chip industry insider told the press that Nvidia has been promoting the specialization of chips to improve performance and power consumption. The GPUs now used for AI computing have structures like Tensor Cores (tensor processing units), and perhaps such structures will be more prevalent in the future. Chen Wei, Chairman of Qianxin Technology, told the press that he speculates there may be specialized GPUs for large model applications in the future, sacrificing some of the original support for display capabilities to support larger matrix calculations. In addition, GPUs are also improving themselves with various technologies, such as adopting more advanced packaging and integration technologies to reduce interconnect power loss and using in-memory computing technology to improve energy efficiency.
As for whether the industry will move towards other AI chips more suitable for large models in the future, or continue to improve on the basis of GPUs, Chen Wei told the press that currently, both forces are in contention. On one hand, there is a demand for new structures and more powerful computing power, and on the other hand, Nvidia already has a mature CUDA ecosystem, with new and old forces rising and falling.
Owen Rogers told the press that in addition to ASICs and GPUs, new SoC (System on a Chip) designs may also emerge in the future, integrating different types of processors, memory, and interconnect technologies to meet the needs of different scenarios for different chips. Enterprises will choose the most suitable AI chips based on their own needs.
Leave a Comment